The Indy Autonomous Challenge (IAC) is a race series that gathers global university teams to compete in challenges to advance technology that can speed the commercialization of fully autonomous vehicles and deployments of advanced driver-assistance systems (ADAS), to increase safety and performance.
The vehicles participating in the IAC are SAE level 4 autonomous, as they are capable of completing circuit laps and overtaking maneuvers without any human intervention. Graham Heeps, along with IAC insiders, in an exclusive feature first published in the April 2024 issue of ADAS & Autonomous Vehicle International, outline the new technologies emerging in autonomous racing and assess their safety, and performance.
It’s more than three years since AAVI reported on the then-new Indy Autonomous Challenge (IAC). Since then, the competition has begun to make good on its mission to prove the safety and performance of
AV technologies in a demanding race environment, solve edge case scenarios, grow new student engineering talent and build some much-needed goodwill for autonomous technologies among the general public.
Races have expanded from no-right-turn US ovals to the more demanding road course of Monza in Italy and, new this year, the famous, partially tree-covered hillclimb at the Goodwood Festival of Speed. Along the way, maximum speeds have increased to 290km/h, overtaking moves are no longer confined to low-risk straightaways and the competing university teams have advanced their AI drivers to the point where IAC needed to build a new car.
Enter the AV-24
Work on the new AV-24 machine began last summer. The AV-21’s Dallara carbon-fiber chassis and Honda-derived engine have received new brake- and steer-by-wire systems to better handle the demands of road course racing. Another major area of improvement relates to reducing the quantity and improving the reliability of the copious onboard wiring and connectors.
“That continues to be an area that needs to evolve even further, because automotive-grade connectors for some of the equipment that you need – network switches, wireless modems, etc – are still hard to source,” explains IAC president Paul Mitchell.
These measures have also cut the car’s weight, as has removing as many as possible of the control units that went with individual sensors: “The feeds from sensors or other systems are not getting processed by a black box from the supplier,” Mitchell says.
“Rather, we have a direct feed into the central computer and let the teams use the raw data coming from the lidar or radar, for example. They can pick and choose how they want to
process it and use it.”
The sensor stack is heavily revised. Out go the AV-21’s three Luminar Hydras, to be replaced by four Volvo EX90-style Luminar Irises – including a rear-facing unit that will help with perception during overtaking moves on road courses. The two 4D radars are also new, with Continental ARS 548 RDIs now on board.
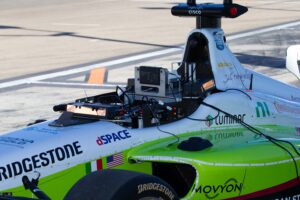
“The new point cloud radar sensor will enable us to do things like visual odometry with the radar point clouds thanks to the embedded speed information,” says C K Wolfe, a technical program manager at the University of California, Berkeley, who leads the simulation and vehicle dynamics subteam for AI Racing Tech (ART). “The different configuration of the lidars, with a larger field-of-view and rear lidar sensor, allows for more interesting algorithms on the sensor fusion side in terms of detecting and classifying opponents or developing your vision stack to navigate with agents overtaking from behind. The upgrades have incorporated a lot of feedback from the different teams for the things that we want to see, to get the edge-case performance that we want out of the vehicles.”
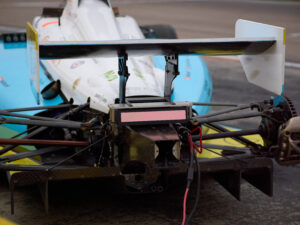
Aside from Berkeley, ART incorporates students from California’s UC San Diego, Carnegie Mellon in Pennsylvania
and the University of Hawai’i Maui College. It was the first team to volunteer an AI test driver for the AV-24, beginning with shakedown sessions at Lucas Oil Indianapolis Raceway Park in November 2023.
With six Allied Vision Mako G-319C cameras and four VectorNav VN-310 GNSS antennas alongside its lidars and radars, the new sensor stack provides a wealth of information to the AI drivers. Wolfe says that the exact algorithm used in competition will differ depending on the track and the conditions on race day.
“Having redundancy in these systems is helpful because this is an expensive, completely custom-built race car,” she notes.
“From a research perspective, you can try out different approaches in terms of the computer vision stack and choose what you want to use. You can also validate the accuracy of the different systems against each other. What we run in the race may be a leaned-down version of that stack. We may just do a camera-lidar projection for the depth and vision piece or run segmentation and then just sample the lidar points within certain areas where we know the other agent is.
“On a road course, the features are different from what you would see at an oval,” she continues.
“In the past when we had the side radar sensors, we ran a wall-detection algorithm. The environment is repetitive on an oval and you know where the wall is going to be. But a road course such as Monza or Putnam Park [in Indiana] has varied features, so you have to take the environment into account when you’re dealing with the perception stack. The way I’m determining where the chicanes are, or how I find those track edges may differ depending on the context of the problem or how I’m trying to approach vision for that track.”
Simulation boost
IAC’s nine teams have been hard at work adapting their AI drivers to the possibilities of the new sensor stack and increased on-track competition, with IAC aiming to get three or more cars together on track as soon as possible.
“As the rules for the competition get less constrained and we see more agents against each other on the track, the problem gets more complicated but also more interesting,” says Wolfe. “You’re introducing high-level approaches to game theory. When we develop our software stack, we talk about neurosymbolic algorithms: neural in the sense that you have an AI driver that has different components and real-time decision making abilities that are more flexible to different environments; and symbolic because you have traditional methods with assurances baked in to the way that you’re making decisions. The goal is to build robust hybrid systems, where you can have flexible decision making at the same time as robust safety assurances.”
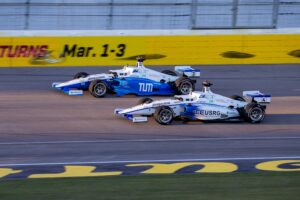
As with any AV development, but especially given the US$1m+ value of each AV-24, simulation is key to the process, enabling ART and the other teams to take risks that they can’t take in the real world.
“You need to test for as many edge cases as possible,” Wolfe confirms. “The important piece is validation in simulation and then the sim-to-real transfer. In simulation, we can get a lot of redundant assurances and go through a full gamut of agent behavior, reconfigure, test for edge cases, tune parameters and safety constrain that behavior.
“We collaborate across the BerkeleyLearnVerify group to leverage and develop edge case simulation testing and validation toolsets. We’re then taking those control, perception and localization algorithms and running them at over 150mph [240km/h] against each other. If you can guarantee safety in these extreme conditions, that very easily translates down into the safety of commercial passenger autonomy. If you can validate system performance for the most difficult problems, it makes
the day-to-day issues trivial.”
An important new addition to the simulation toolbox for all the IAC teams – and a pragmatic stepping stone to increasing the on-track car-count in IAC competitions – arrives in 2024. Series sponsor dSpace has created the Simphera software-in-the-loop setup such that teams will be able to compete in virtual races with high-fidelity models, either locally on a PC or in the cloud. Opponents will be either generic traffic cars, multiples of the team’s own AI drivers or, by arrangement, other teams.
“Our goal is to create races in the cloud…we are fine-tuning the vehicle models so they will behave exactly aas the real car,” says Raimund
Sprick, senior manager, automated driving and software solutions, dSpace. “We are fine-tuning the vehicle models so they will behave exactly as the real car, and recreating the
track at Monza. Each team will have its own workspace where it can upload its controller and run the test in the cloud. The advantage there is that we can run several simulations in parallel.”
“The real edge that they’re giving us is the ability to race against other teams and get an insight into how the other teams make these decisions,” comments ART’s Wolfe. “Being able to get that insider information and test your software against the other teams instead of only in your own validation pipeline or maybe benchmarking against yourself, you’ll see how you measure up against the others before we get to the competition cycle. It will be a very interesting twist in the way that people develop or try to predict what their opponents are going to do.”
The next step
As part of its ongoing research, the AI Racing Tech team is exploring neurosymbolic high-level planning for the agent– learning-based approaches for learning high-level planning and behavior as well as parameter learning from the controls perspective. Says Wolfe, “This research approach allows for cross-platform-transferable autonomy. You can also learn the vehicle dynamics parameters of the agent in real time and then feed them back into your system. That way you can have better-tuned control systems for the agent behavior.”
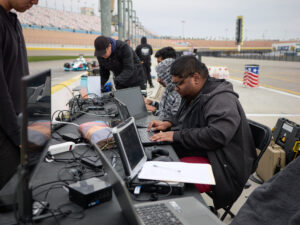
This could include exploiting tire models to feed information about the current behavior of the car on track into the AI driver’s decision making process. The problem, she says, is the “closed- source” nature of motorsport, which makes it hard to get the data in the first place – such as detail on how changes in the friction coefficients as the tires heat up affect the car’s behavior.
“We want to be able to determine from the behavior of the car what those parameters are and feed them back into how we’re making decisions in our control stack,” Wolfe explains. “Dealing with how the car responds to the track conditions has become a competitive edge. Humans have a feel for how the car moves and behaves but it’s difficult to program a car to have that same intuition for how the system behaves. You can take data from
real race car drivers and there’s a little bit of behavioral cloning in the way that you’re looking at executing the software stack, but the conditions are very varied and different. There’s no way to update it to understand the entire gamut of what that behavior should look like.
“Determining some of these things in real time and trying to feed that back into the system for more intelligent decision making is an active research area for us and, I think, for all the teams,” she concludes. “Writing and optimizing code that can run in real time, that can inference fast enough for the driver and the behavior to react quickly enough, is a huge challenge.”